
EYES IN THE SKY
New Course for Spring 2021
Nicholas School of the Environment
Duke University
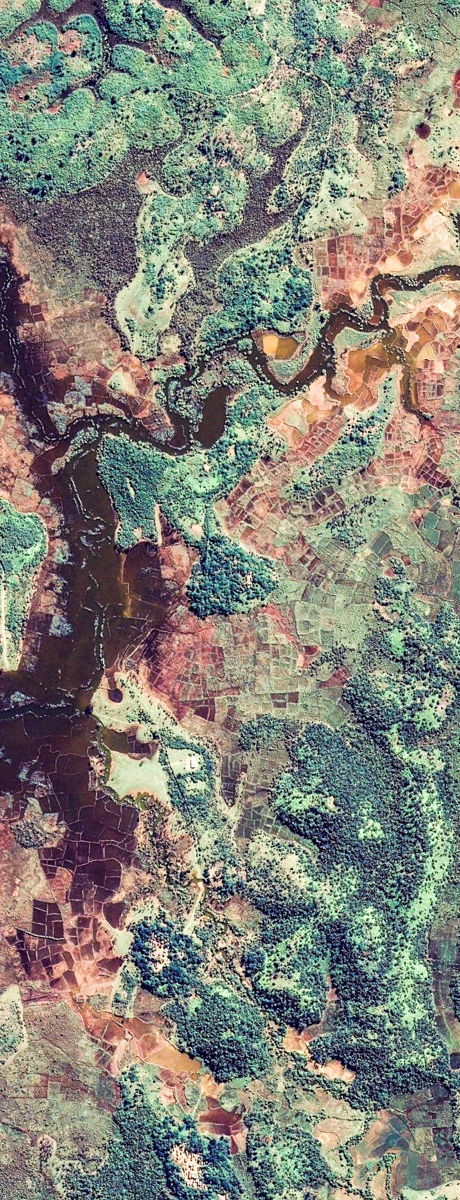
EYES IN THE SKY | REMOTE SENSING FROM SATELLITES TO DRONES
Synopsis: This course spans current and emerging remote sensing applications for ecology and conservation, with in-depth treatment of satellite and drone applications in coastal biological and ecological research through client-based group research projects. Comprehensive exploration of current platforms and sensor technologies (e.g. multispectral, hyperspectral and thermal imaging), theoretical and technical foundations of remote sensing (e.g. georectification, image analysis, landscape classification) and broad exposure to practical applications such as animal detection, land cover mapping, digital elevation models, change analysis and essential ground truthing techniques for both drone and satellite remote sensing products. Experiential work focused on applying remote sensing workflows to real world questions and customization of approaches to different remote sensing platform/sensor combination for ecological or conservation applications.
Collaborative client project: Groups of students will engage with a natural resource agency or NGO to help them study or manage an environmental problem tractable using drone and satellite-based remote sensing.
OVERVIEW
Spring 2021
1. Discovery
Foundational Learning
Electromagnetic spectrum
Atmospheric effects
Aerodynamic and astrophysical constraints
Computation Approaches
Exploration of remote sensing bands, comparisons amongst platforms
Experiential Learning
[virtual] Hands-on with sensors, platforms
Demonstrations of thermal and multi/hyperspectral imaging
UAS remote sensing demonstrations
Group work
Group into team exercises
Introduction to client/projects
Project scoping
2. Design
Foundational Learning
Change detection
Habitat classification
Computational Approaches
Machine learning
Structure from motion
Supervised and unsupervised classification
Experiential Learning
[virtual] Team build a research grade drone
Program for automated flight
EO, thermal and multispectral data collection
Group work
Execute data collection
Execute initial data analysis
3. Deliver
Foundational Learning
Developing strong inference
Understanding and communicating uncertainty
Risk and decision making
computational approaches
Decision tree analysis
Statistical assessments
Defining and describing relevant change
Experiential Learning
Mock presentation through role playing exercise
Group work
Creation of final products
Presentation of final products to clients (presentation, web-based visualizations, )
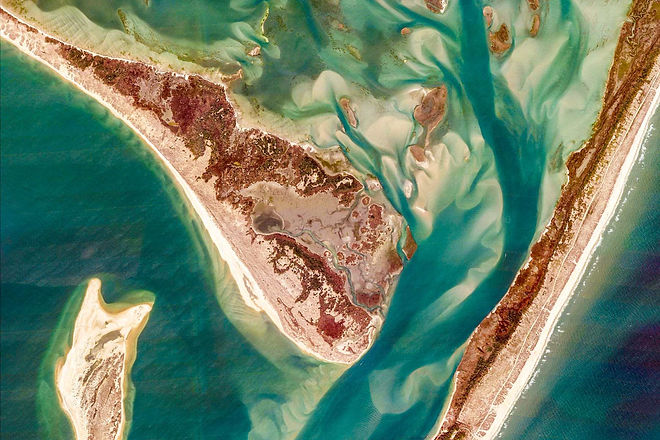
CLIENT ORIENTED COLLABORATIVE GROUP PROJECT
SYLLABUS
Course Level: 500 level
Undergraduate codes: Seminar, STS, QS, NS
Semester: Spring 2021
Audience: juniors, seniors, masters, doctoral
Credits: 3
Prerequisites:
This course is aligned along four complimentary learning axes:
Foundational Learning: course components that address key theories, physical laws and core facts
Computational Approaches: course components that address basics of working with remote sensing data via a number of computational workflows
Experiential Learning: course components that integrate foundational and computational learning through hands-on experiences
Group work for clients: course components that revolve around fostering success in group projects, collecting/retrieving data, and conducting remote sensing analyses, and producing final products and interacting with clients.
Learning Objectives. After successfully completing this class students will be able to:
Define basic concepts of remote sensing as they are applied to ecology and conservation
Describe how satellite and drones are used to study the animal detection, land cover mapping, digital elevation models, change analysis
Identify specific remote sensing platforms/sensors and the data/products that are produced by them
Compare and contrast drone and satellite-based remote sensing approaches
Create and fly a drone remote sensing system
Design spatial sampling protocol for drone imagery capture
Apply specific satellite and drone remote sensing workflows to ecological and conservation projects
Understand costs of drone technology in terms of economic limits of different clients/users
Plan projects, analyze data, and assemble inference regarding remote sensing products for a client seeking to solve an ecological or environmental problem
Demonstrate collaborative ability within teams and with client
Communicate analysis methods and outcomes for client throughout the project